UC Irvine study finds mismatch between human perception and reliability of AI-assisted language tools
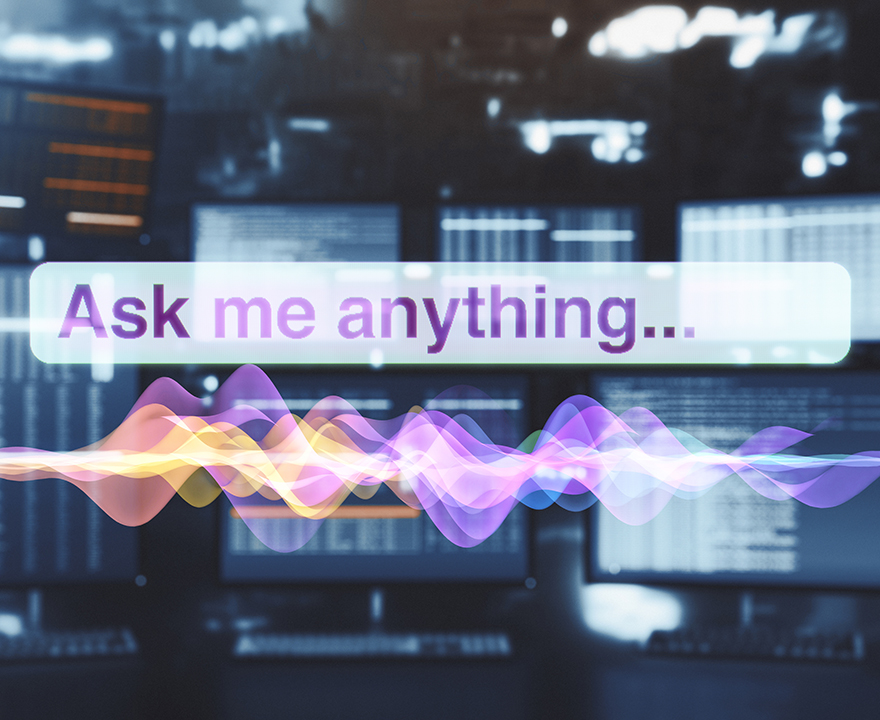
UC Irvine study finds mismatch between human perception and reliability of AI-assisted language tools
- January 22, 2025
- Adding uncertainty phrasing to large language model responses can help users better gauge accuracy
-----
As AI tools like ChatGPT become more mainstream in day-to-day tasks and decision-making
processes, the ability to trust and decipher errors in their responses is critical.
A new study by cognitive and computer scientists at the University of California,
Irvine finds people generally overestimate the accuracy of large language model (LLM)
outputs. But with some tweaks, says lead author Mark Steyvers, cognitive sciences
professor and department chair, these tools can be trained to provide explanations
that enable users to gauge uncertainty and better distinguish fact from fiction.
“There’s a disconnect between what LLMs know and what people think they know,” says Steyvers. “We call this the calibration gap. At the same time, there’s also a discrimination gap – how well humans and models can distinguish between correct and incorrect answers. Our study looks at how we can narrow these gaps.”
Findings, published online in Nature Machine Intelligence, are some of the first to explore how LLMs communicate uncertainty. The research team included cognitive sciences graduate students Heliodoro Tejeda, Xinyue Hu and Lukas Mayer; Aakriti Kumar, ’24 Ph.D.; and Sheer Karny, junior specialist. They were joined by Catarina Belem, graduate student, and Padhraic Smyth, Distinguished Professor and director of the Data Science Initiative from computer science.
Currently, LLMs - including ChatGPT - don’t automatically supply language in responses that indicate the tool’s level of confidence in its accuracy. This can mislead users, says Steyvers, as responses can oftentimes appear confidently wrong.
With this in mind, researchers created a set of online experiments to provide insight on human and LLM perception of AI-assisted responses. They recruited 301 native English-speaking participants in the U.S., 284 of whom provided demographic data, resulting in a split of 51% female, 49% male and a median age of 34. Participants were randomly assigned sets of 40 multiple choice and short-answer questions from the Massive Multitask Language Understanding dataset - a comprehensive question bank ranging in difficulty from high school to professional level, covering topics in STEM, humanities, social sciences and other fields.
For the first experiment, participants were provided default LLM-generated answers to each question, and they had to decide the likelihood that the responses were correct. The research team found that participants consistently overestimated the reliability of LLM outputs; standard explanations did not enable them to judge the likelihood of correctness, leading to a misalignment between perception and reality of the LLM’s accuracy.
“This tendency toward overconfidence in LLM capabilities is a significant concern, particularly in scenarios where critical decisions rely on LLM-generated information,” he says. “The inability of users to discern the reliability of LLM responses not only undermines the utility of these models, but also poses risks in situations where user understanding of model accuracy is critical.”
The next experiment used the same 40-question/LLM-provided answer format, but instead of a singular, default LLM response to each question, the research team manipulated the prompts so that each answer choice included uncertainty language that was linked to the LLM’s internal confidence. Phrasing indicated the LLM’s level of confidence in accuracy – low (“I am not sure the answer is A”), medium (“I am somewhat sure the answer is A”) and high (“I am sure the Answer is A”) – alongside explanations of varying lengths.
Researchers found that providing uncertainty language strongly influenced human confidence. Low confidence LLM explanations corresponded to significantly lower human confidence in accuracy over those marked by the LLM as medium, with a similar pattern emerging for medium vs. high confidence explanations.
Additionally, the length of the explanations also affected human confidence in the LLM answers. Participants had higher confidence in longer explanations over shorter ones, even when the extra length didn’t improve answer accuracy.
Taken together, the findings underscore the importance of uncertainty communication and the effect of explanation length in influencing user trust in AI-assisted decision-making environments, says Steyvers.
“By modifying the language of LLM responses to better reflect model confidence, users can improve calibration in their assessment of LLMs’ reliability and are better able to discriminate between correct and incorrect answers,” he says. “This highlights the need for transparent communication from LLMs, suggesting a need for more research on how model explanations affect user perception.”
Funding for this work was provided by the National Science Foundation under award 1900644.
-Heather Ashbach, UCI Social Sciences
-pictured top to right: AI chat tool (by dem10/iStock); Mark Steyvers in office (by
Steve Zylius/UCI).
-----
Would you like to get more involved with the social sciences? Email us at communications@socsci.uci.edu to connect.
Share on:
connect with us: